Autonomous AI Labs Upend the Pace of Discovery
The April 17, 2025 episode of FYI — For Your Innovation features Geoffrey von Maltzahn detailing how Lila couples AI, proprietary data, and fully automated laboratories to build “scientific super‑intelligence.”
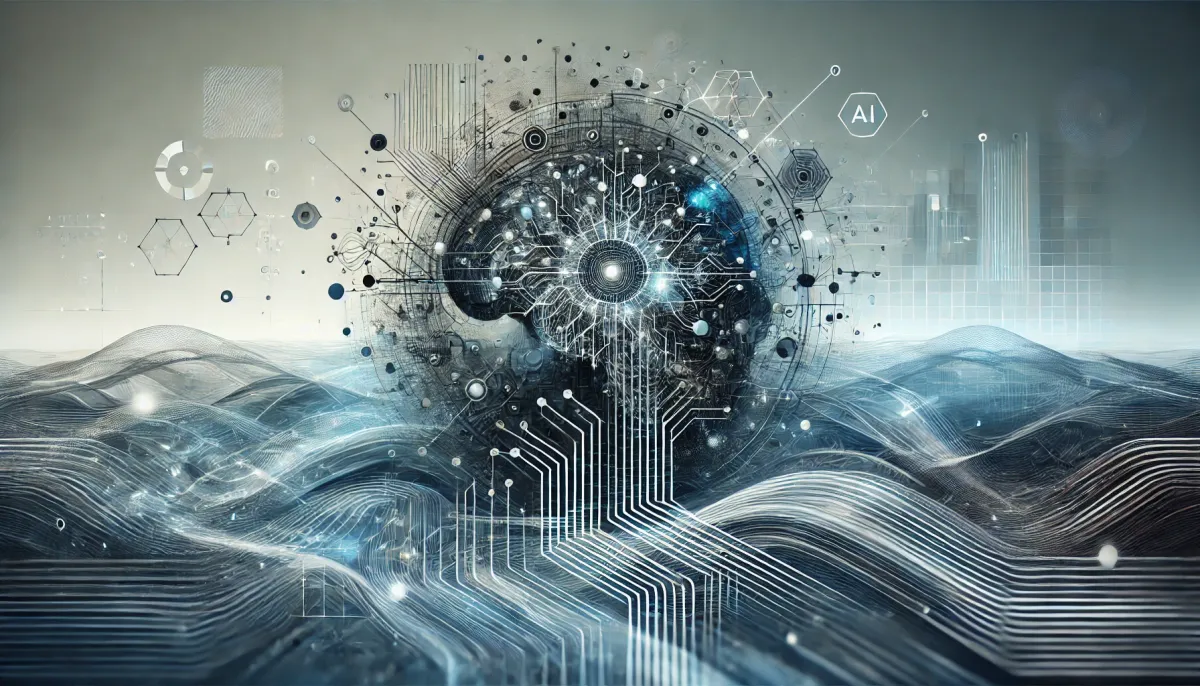
- My 'briefing notes' summarize the content of podcast episodes; they do not reflect my own views.
- They contain (1) a summary of podcast content, (2) potential information gaps, and (3) some speculative views on wider Bitcoin implications.
- Pay attention to broadcast dates (I often summarize older episodes)
- Some episodes I summarize may be sponsored: don't trust, verify, if the information you are looking for is to be used for decision-making.
Summary
The April 17, 2025 episode of FYI — For Your Innovation features Geoffrey von Maltzahn detailing how Lila couples AI, proprietary data, and fully automated laboratories to build “scientific super‑intelligence.” He explains early proofs - triple‑efficiency mRNA constructs and ultra‑cheap hydrogen catalysts -achieved by tiny teams in months, not years. The conversation previews governance, safety, and commercialization challenges as autonomous science scales across domains.
Note: while this is a non-Bitcoin episode, I have it posted here as I think there are important implications for Bitcoin.
Take-Home Messages
- Data Advantage: Proprietary experimental datasets, not public web text, become the decisive moat for AI‑driven R&D.
- Acceleration Proof: AI‑designed mRNA and catalysts show months‑not‑years breakthroughs, validating the autonomous‑lab model.
- Safety Levers: Closed‑loop laboratories enable mass‑balance tracking and real‑time intervention, but oversight must keep pace.
- Flexible Monetization: Platform access, partnerships, and spin‑outs fund growth while preserving focus on core scientific intelligence.
- Policy Sprint: Regulators must update IP, bio‑risk, and access frameworks now to harness benefits and curb misuse.
Overview
Geoffrey Von Maltzahn presents Lila’s mission to build scientific super‑intelligence by automating every step of the scientific method. He argues public‑data foundation models plateau without proprietary feedback loops, making autonomous labs the new edge. Seven “science factories” launching this year will industrialize hypothesis testing across biology, chemistry, and materials.
Early demonstrations underscore the acceleration effect. A three‑person team used the platform to create mRNA sequences that triple protein output versus leading COVID‑19 vaccines in four months. In materials science, AI identified earth‑abundant catalysts 1,000‑5,000 × cheaper than platinum group metals for green‑hydrogen electrolysis—results experts expected would take a decade.
Safety remains central. Von Maltzahn stresses that closed‑loop labs allow containment and monitoring impossible in open‑model releases, yet objective‑function alignment and regulatory verification are unfinished work. He invites policymakers to treat safety tooling as integral infrastructure, not an afterthought.
Commercially, Lila prefers platform access and downstream partnerships over in‑house product pipelines, keeping capital focused on expanding intelligence. This model could blur disciplinary boundaries, shifting scientists from manual bench work to high‑level hypothesis generation and rapid model refinement.
Stakeholder Perspectives
- Regulators: Demand verifiable safety protocols and updated IP statutes before broad deployment.
- R&D‑Intensive Corporations: See faster discovery but worry about dependence on proprietary data silos.
- Academic Institutions: Face reskilling pressure as manual experimentation declines and cross‑domain work expands.
- Investors: Value data moats and scalability, tempered by commercialization timing and regulatory risk.
- Low‑Income Countries: Seek equitable access to prevent a widening scientific intelligence divide.
Implications and Future Outlook
Autonomous science platforms can compress multi‑year discovery cycles into quarters, redrawing competitive lines in therapeutics, energy, and advanced materials. Governments that modernize safety standards, data‑sharing incentives, and IP rules will attract talent and investment, while laggards risk scientific marginalization.
Energy and resource demands of large‑scale AI labs rise sharply. Efficiency benchmarks, circular material flows, and renewable sourcing strategies must accompany expansion to avoid environmental backlash that could stall deployment.
Access equity is critical. Tiered platform models, open‑data offsets, and international cooperation can democratize participation, ensuring accelerated discovery fuels inclusive growth rather than concentrated advantage.
Some Key Information Gaps
- How can regulators verify safety without slowing research velocity? Rapid, scalable oversight tools are vital to balance innovation with bio‑risk containment.
- Which metrics ensure AI scientists pursue societally aligned goals? Robust alignment research prevents resource misallocation and harmful outcomes.
- How can lower‑income regions gain affordable access to autonomous science? Equitable participation averts a widening intelligence divide and fosters global resilience.
- How should IP law evolve to recognize AI‑generated discoveries? Legal clarity underpins investment, collaboration, and commercialization across sectors.
- What energy‑efficiency standards are necessary for AI science factories? Early sustainability benchmarks safeguard scalability and align with climate goals.
Broader Implications for Bitcoin
Decentralized Data vs. Proprietary Silos
Autonomous labs’ reliance on private datasets mirrors debates over centralized versus decentralized ledgers. Blockchain‑based science cooperatives could emerge to counterbalance data monopolies, echoing Bitcoin’s role in financial decentralization and challenging incumbents to share or tokenize experimental outputs.
Energy‑Market Innovation and Bitcoin Mining
Ultra‑cheap electrocatalysts and accelerated materials discovery may lower green‑hydrogen and renewable‑storage costs, indirectly benefiting Bitcoin miners seeking low‑cost, sustainable power. Faster invention cycles could reshape energy‑arbitrage strategies that underpin off‑grid mining operations.
Hardware Supply Chains and ASIC Design
Rapid materials breakthroughs can feed directly into semiconductor innovation, potentially yielding more efficient ASICs for Bitcoin hashing. Nations with autonomous‑lab capacity may secure early access to novel substrates, tightening control over critical mining hardware supply chains.
Governance Lessons for Permissionless Networks
Objective‑function alignment challenges in AI labs parallel consensus‑rule risks in decentralized networks. Bitcoin’s layered governance—open review, broad node participation, and cautious upgrades—offers templates for balancing innovation speed with systemic safety in autonomous science.
Comments ()