Navigating AI Value Capture: Cost Savings vs. Revenue Generation
In a Sept 4, 2024 ARK Invest podcast, team members discus the emerging dynamics of value capture in the AI ecosystem, primarily contrasting cost savings against revenue generation as pathways for AI monetization.
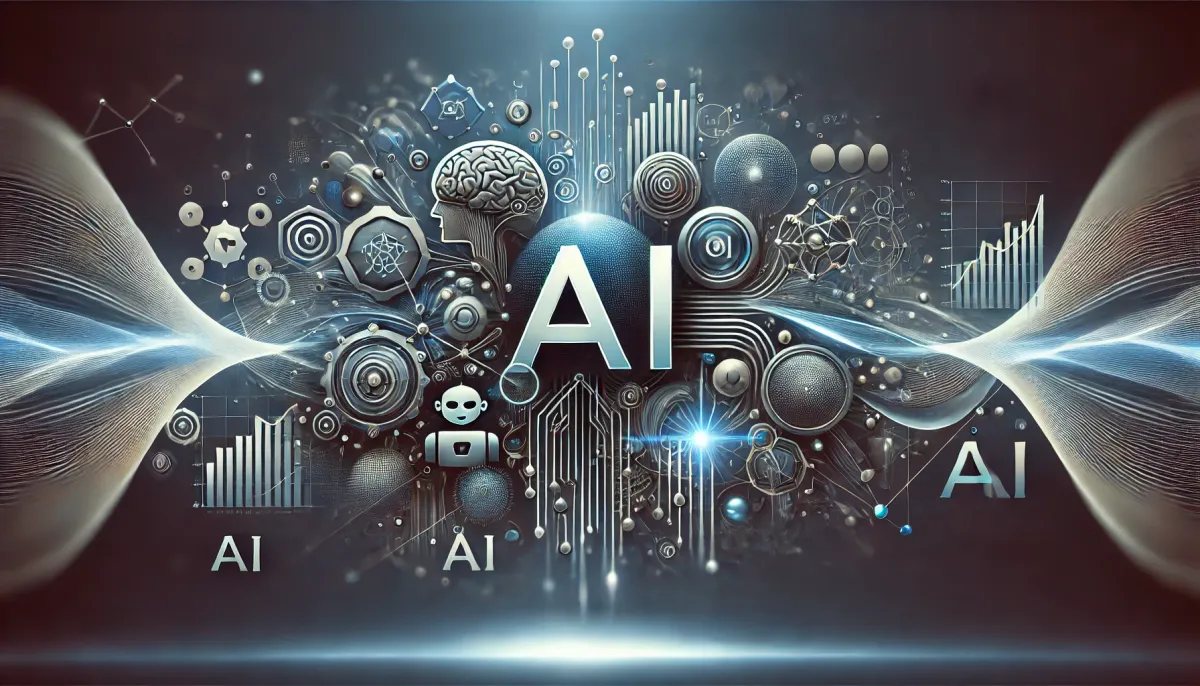
Summary
This podcast episode examines the contrasting approaches of cost savings and revenue generation in AI monetization, highlighting that revenue-linked models provide clearer value capture for providers. As AI technologies evolve, performance-based pricing and niche market strategies are seen as key to sustaining AI’s financial impact.
Take-Home Messages
- Cost vs. Revenue Models: Revenue-focused AI models offer clearer monetization pathways than cost-saving approaches, making them more attractive for providers.
- Performance-Based Pricing: Attaching pricing to AI performance outcomes is emerging as a strategic approach to defend value capture in competitive markets.
- Commoditization Challenge: The rapid replication of AI technologies leads to commoditization, necessitating differentiation beyond just technical capabilities.
- Niche AI Applications: AI solutions targeting niche markets present untapped opportunities, allowing companies to customize offerings and secure unique market positions.
- Strategic Integration: Integrating AI into revenue-generating activities rather than merely cost-saving initiatives will be critical for companies aiming to sustain competitive advantages.
Overview
The podcast delves into the complexities of AI value capture, contrasting cost savings against revenue generation as key monetization strategies. Using Amazon's internal AI deployment as a case study, the hosts discuss the significant operational efficiencies achieved, such as saving thousands of developer years and considerable costs. However, the monetization of such cost savings proves less straightforward, as the value primarily accrues to the end-user rather than the provider.
The discussion emphasizes that revenue generation models, which tie monetization directly to new income streams, offer a clearer path for value capture. The hosts cite examples like the Apple App Store, where providers take a cut of generated revenue, demonstrating a more defensible and sustainable business model. This approach is particularly relevant in the AI space, where rapid replication and commoditization of technologies present ongoing challenges to maintaining pricing power.
The conversation also explores how AI's accessibility lowers barriers to entry, intensifying competition and threatening traditional business models. As AI tools become more ubiquitous, differentiation based on performance guarantees and targeted, niche applications becomes essential. The episode concludes by highlighting that successful AI monetization will require aligning AI solutions with core revenue-driving activities, ensuring that value capture is not only defensible but also scalable.
Broadcast Highlights
- Amazon's AI deployment saved thousands of developer years, showcasing AI's impact on operational efficiencies.
- Monetizing cost savings remains a challenge for AI providers, favoring revenue-focused models for clearer value capture.
- High capital expenditures on AI infrastructure, such as Nvidia's chip sales, underline the need for clear ROI justification.
- Revenue generation models like the Apple App Store offer more straightforward monetization pathways.
- The commoditization of AI technologies poses significant risks to pricing power and market differentiation.
- Performance-based pricing models align AI monetization with measurable outcomes, enhancing defensibility.
- The ease of deploying AI technologies intensifies competition by lowering market entry barriers.
- Differentiating AI applications between enterprise and consumer markets helps tailor effective monetization strategies.
- AI solutions in niche markets represent opportunities for customized, high-value applications.
- Future AI success will depend on integrating AI into core business strategies that drive new revenue streams.
Implications
The insights from this podcast suggest that companies aiming to capitalize on AI need to focus on revenue-generating models that directly link value capture to performance outcomes. This shift not only provides a defensible business model but also ensures that AI investments yield measurable returns. The rapid commoditization of AI tools requires companies to differentiate their offerings through unique applications and performance guarantees, particularly in niche markets.
As AI technologies continue to evolve, integrating these solutions into core business strategies that drive revenue will become increasingly important. This approach will allow companies to maintain competitive advantages, navigate the challenges of commoditization, and capitalize on the expanding potential of AI to reshape industries.
Future Outlook
The trajectory of AI monetization will likely favor models that tie value capture directly to revenue generation. Performance-based pricing and niche market strategies will be essential for companies to sustain competitive advantages and secure unique positions in a crowded market. To steer toward a more successful future, stakeholders must focus on integrating AI into revenue-generating activities and continuously innovating to address the evolving dynamics of AI-driven markets.
Information Gaps
- Monetization of AI Efficiencies: Identifying viable business models to monetize AI-driven operational efficiencies remains a critical research need.
- Defensibility in AI Products: Exploring strategies beyond technical differentiation, such as leveraging proprietary data, is essential for building defensible AI offerings.
- Performance-Based Pricing: Developing optimized performance-based pricing models for AI could enhance value capture and attract broader enterprise adoption.
- AI in Niche Markets: Investigating the potential of AI-driven solutions in niche markets offers new avenues for customized applications and growth.
- Balancing AI Applications: Understanding the distinctions between enterprise and consumer AI applications can guide more strategic product development and marketing.
Comments ()